TLDR
Life is too short for exact matches!
In this video I will build out a Tableau Prep flow that demonstrates one approach to perform fuzzy matching. We’ll perform the fuzzy matching technique on two lists of companies. One list contains a standardized list and the other contains a non-standardized list, and we will match them together using Tableau’s fuzzy algorithm.
Intro
As a working data professional, one of my main responsibilities is to ensure the accuracy and consistency of the data that I work with. One of the challenges I face is dealing with different variations of company names that may exist in different datasets. In the video above, I walk you through a fuzzy match join that I recently performed using Tableau Prep, a data preparation tool, to reconcile these variations.
What is Fuzzy Matching?
First, I want to introduce you to the concept of fuzzy matching. It’s a technique used to match data when there are slight differences in how the data is presented (most likely as a result of bad data governance). For example, if you have two data sets with company names, one may list a company as “Apple Inc.” while the other may list the same company as “Apple Incorporated.” Fuzzy matching would help you match these two records, even though the names are slightly different.
In Tableau Prep, unfortunately fuzzy matching is not a straightforward process like it is in Excel or other tools like Power BI or Alteryx. However, we can use a workaround to achieve a somewhat similar result. Tableau Prep allows you to automatically group values together using fuzzy-match algorithms that find similar values.
High Level Flow Process
To get started, let’s say we have two lists of companies. One is a master list of companies that we want to use as our reference, or “golden” copy. The other is a list of companies that may be misspelled or unstandardized. We want to join these two lists together using fuzzy matching (although technically we employ fuzzy grouping options to enable traditional matching).
Within Tableau Prep I start with two groups of data, a clean “golden copy” of company names and a list of company names that contain poor data quality (i.e., user-entered data, which may have misspelled or unstandardized company names).
Once we have imported both data sets, we can append them using a union step. The union will combine the two lists of company names into one.
Next, we need to perform a fuzzy algorithm on the list of company names. Remember that both the golden copy and the misspelled names are stacked into one column. In Tableau Prep, we’ll use the fuzzy grouping capability to group together similar values, even if they’re not exact matches. We’ll use this technique to group the misspelled company names with their correct counterparts in the “golden” copy.
Granted this approach does not scale terribly well over a large dataset because we need to eyeball each grouping to ensure it is acceptable, but it is a good start. I don’t know of any fuzzy algorithm that guarantees 100% results, as “fuzzy” is inherent in the name of the approach.
Here are a couple of must read posts from the Tableau Knowledge Base for Fuzzy Grouping if you want to understand these automated grouping options:
- Automated Grouping in Tableau Prep Builder
- Search for this section: Automatically map values to a standard value using fuzzy match
After the values in the combined column are grouped together into a standardized clean format, we simply need to deduplicate our values so we are left with a 1 to 1 mapping between the incorrect data and the cleaned up result of the fuzzy grouping. We can use this “translation table” as a means to join our Golden Copy dataset to the less standardized dataset (which has the sales data we need to analyze).
Result
By using fuzzy match and Tableau Prep, I was able to successfully reconcile the variations in the company names and match the sales quotes in the “poor data quality” company names data set to their corresponding IDs in the clean golden copy names data set. If you’re stuck in this kind of a scenario at work, use this process to advocate for a cleanup of the data quality at the source of entry or creation.
In conclusion, fuzzy matching is a powerful technique that can help you match data even when the data may not be spelled correctly or may have slight variations. Tableau Prep may not have a straightforward fuzzy matching feature (a la Excel), but we can use fuzzy grouping to achieve similar results.
Here is a pic of the flow we build in the video. I add additional steps for this unique case but ideally to perform the fuzzy grouping we could stop much earlier in the process.
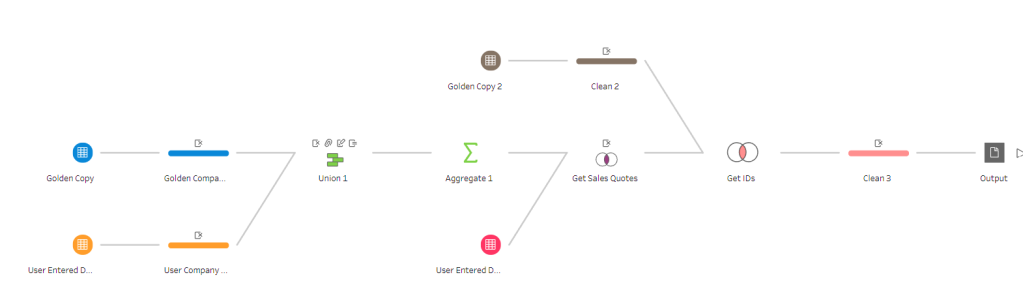
Additional “Fuzzy” Videos (Not Quality Wise, Topic Wise)
- If you need to know how to perform Fuzzy Matching in Excel, watch this video.
- Address Matching in Excel Using Levenshtein Distance
I appreciate everyone who has supported this blog and my YouTube channel via merch. Please check out the logo shop here.
Stay in contact with me through my various social media presences.
Thank you!!
Anthony B Smoak